


Now let’s see how to use SAS’ new Support Vector Data Description (SVDD) procedure to find the ‘smoking gun’.

Identify Asset Degradation and Unstable Operation using Support Vector Data Description (SVDD) The following video showcases common JMP tools used to explore and identify asset degradation. I like to use JMP for initial explorations. Typically analysts start with visual and descriptive modeling approaches to perform condition monitoring and anomaly detection. We assume that this data represents normal operating conditions. The validation data contains all data for the remaining 188 engines. The training data contains the first 25% of engine cycle sensor measurements for 30 randomly sampled engines. The data contains 218 different engines with end of useful life ranging between 128 and 357 engine cycles. The data contains 26 variables including: engine ID, cycle number, three operational settings, and 21 sensor measurements. The data showcased in this blog is NASA’s 2008 Prognostics and Health Management Challenge Data Set ( PHM08) simulating turbofan engine degradation.
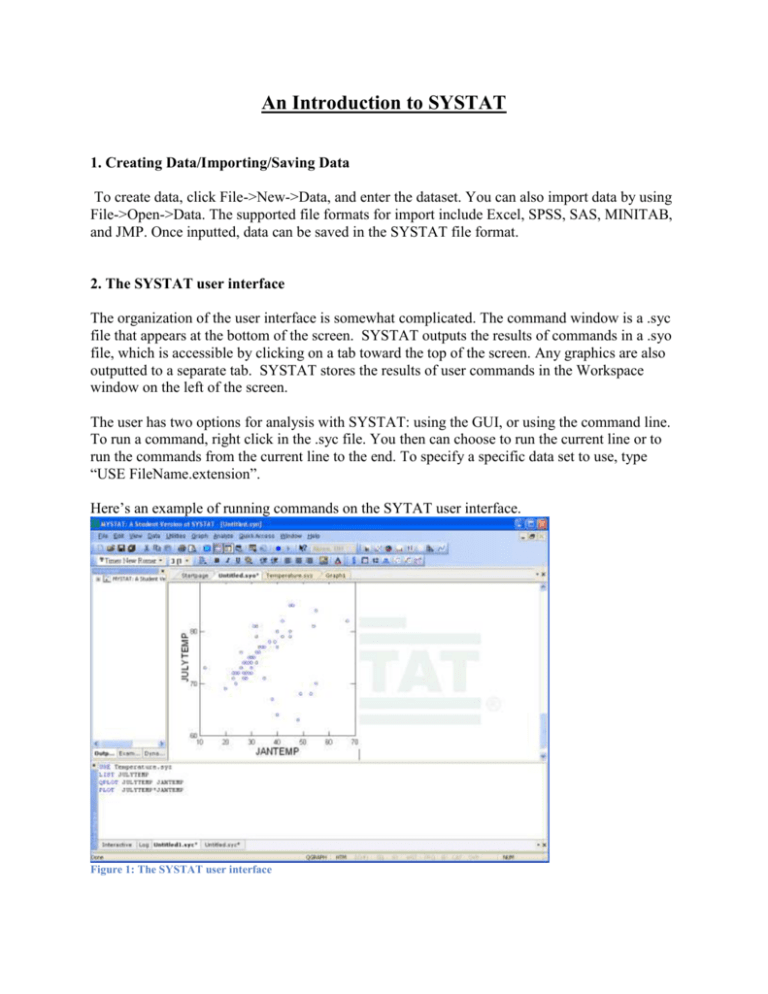
So you can appreciate how slick the new SVDD procedure is, let’s consider typical approaches for condition monitoring and anomaly detection for high-frequency multivariate data. Traditional Asset Degradation Investigation Approaches As of May 23rd, you can export the resulting SVDD score code directly into SAS Event Stream Processing (ESP) to easily detect and alert these system anomalies in near real-time. SAS’ new Support Vector Data Description (SVDD) procedure will speed up your ‘smoking gun’ investigation efforts by generating one output model instead of dozens, if not hundreds, typically created. It wasn’t easy.įortunately SAS’ Advanced Analytics R&D group released several new machine learning algorithms in 17W12 designed for condition monitoring and anomaly detection of high-frequency multivariate data. Have you ever been asked to find the ‘smoking gun’ for a high-cost critical asset failure? What about identifying predictive asset degradation patterns? Were you using high-frequency multivariate data, making the exercise feel more like you were looking for a ‘smoking needle’ in a haystack? I have.
